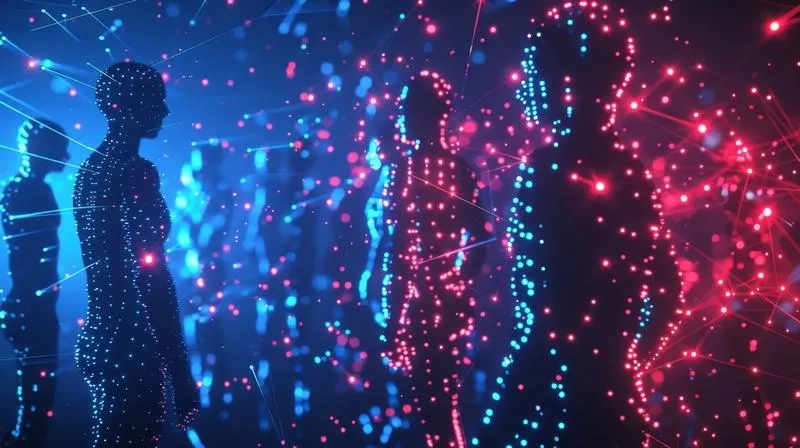
Predictive Policing Gone Wrong – Are AI Algorithms Creating a Self-Fulfilling Crime Crisis
In recent years, predictive policing has emerged as a powerful tool for law enforcement, utilizing AI algorithms to forecast where crimes are most likely to occur or even which individuals may be at risk of criminal behavior. While this technology is designed to make communities safer, it’s sparking debate over its effectiveness and ethical implications. Critics argue that predictive policing can reinforce biases, target vulnerable communities, and ultimately create a self-fulfilling crime crisis.

This article explores the potential dangers of predictive policing, how it operates, and why it may be doing more harm than good.
What is Predictive Policing?
Predictive policing uses machine learning and big data to identify patterns in crime data. By analyzing historical crime rates, social media posts, geographic locations, and individual profiles, these algorithms aim to predict where and when crimes are likely to happen. Police departments can then allocate resources to high-risk areas or monitor specific individuals deemed at risk of criminal behavior.
Common predictive policing tools include:
Place-based algorithms: These predict crime hotspots by analyzing geographic patterns.
Person-based algorithms: These assess individual risk levels based on criminal records, social networks, and other personal data.
Social media monitoring: Some predictive models analyze social media activity to detect potential gang activity or forecast civil unrest.
How Predictive Policing Can Create a Self-Fulfilling Crime Crisis
Although predictive policing is meant to prevent crime, there is evidence that it can contribute to a self-fulfilling prophecy. Here’s how:
Reinforcing Bias in High-Risk Areas: Predictive algorithms often rely on historical crime data, which can be biased due to previous over-policing of certain communities. By sending more police to these areas based on predictive data, law enforcement may catch more minor infractions simply because of increased presence, reinforcing the idea that these areas are high-crime zones.
Targeting Individuals Based on Background: Person-based algorithms can label individuals as “high risk” based on prior offenses, social connections, or residence in a certain neighborhood. This can lead to repeated stops or surveillance of individuals who might otherwise not commit crimes, stigmatizing them and potentially influencing their future actions.
Impact on Community Trust: Communities targeted by predictive policing often perceive this as discrimination, eroding trust between residents and law enforcement. When communities view the police as biased, cooperation decreases, making it more challenging to address actual crime effectively.
Creating a Vicious Cycle of Surveillance and Arrests: Once an area or individual is flagged as high risk, they are more likely to be under surveillance. This increased monitoring can lead to more arrests for minor infractions, reinforcing the data that the area or individual is high-risk, creating a cycle that’s hard to break.
Real-World Examples of Predictive Policing Gone Wrong
Chicago’s Strategic Subjects List: In Chicago, police used an algorithm to rank individuals by their likelihood of committing or becoming victims of violent crime. Many individuals on the list had no criminal records, yet they were monitored based on arbitrary factors like acquaintances or neighborhood. The program was eventually criticized for racial profiling and lack of transparency.
Los Angeles’ PredPol Program: Los Angeles Police Department used a program called PredPol to predict crime hotspots. However, a report by the Brennan Center found that the software often flagged poor and minority neighborhoods, leading to excessive policing in those areas and reinforcing pre-existing biases.
New York City’s Gang Database: NYC’s police force uses algorithms to monitor social media and identify potential gang members. However, many individuals have been added to the database with little evidence, raising concerns about profiling and unjust surveillance.
Why Bias in Predictive Policing is Hard to Avoid
Predictive policing algorithms often mirror the biases present in the data used to train them. Since these algorithms are based on historical crime data, which may reflect past discriminatory practices, they can unintentionally reinforce those same biases. For instance:
Over-Policing of Minority Communities: In cities where minority communities have historically been over-policed, crime data will show higher crime rates in those areas. Predictive algorithms trained on this data will continue to flag these neighborhoods as high-risk, leading to more police presence and arrests, regardless of actual crime levels.
Limited Accountability and Transparency: Many predictive policing tools are proprietary, meaning the algorithms and data are not open to public scrutiny. This lack of transparency makes it difficult to hold developers accountable for potential biases or inaccuracies in the systems.
Ethical and Legal Concerns Surrounding Predictive Policing
Predictive policing also raises ethical and legal issues, including:
Privacy Invasion: Surveillance of individuals deemed “high risk” by an algorithm raises significant privacy concerns, particularly if they have not committed any crime. Constant surveillance can infringe on personal freedom and create a culture of fear in targeted communities.
Potential for Discrimination: By reinforcing existing biases, predictive policing can lead to discriminatory practices. Individuals may be unfairly targeted based on factors like race, neighborhood, or social connections, which are not inherently linked to criminal behavior.
Erosion of Presumption of Innocence: Predictive policing can lead to individuals being treated as potential criminals without evidence. This preemptive approach undermines the legal principle of “innocent until proven guilty” and raises questions about due process.
The Future of Predictive Policing – Finding a Balanced Approach
While predictive policing has serious pitfalls, it also has the potential to be used responsibly. Here are some ways law enforcement could improve its application:
Bias-Free Data Training: By using unbiased data sources or focusing on crime factors rather than demographic information, predictive policing algorithms could reduce discriminatory outcomes.
Transparent Algorithm Development: Making predictive policing algorithms transparent and open to third-party evaluation could help identify and mitigate biases, building public trust in the technology.
Community-Based Policing Strategies: Engaging communities in crime prevention, rather than relying solely on predictive technology, could help address root causes of crime. A balanced approach that combines community input with technology may yield better results.
Regular Audits and Accountability Measures: Law enforcement agencies using predictive policing should be required to conduct regular audits to assess the impact and accuracy of these systems, ensuring that they don’t create disproportionate impacts on specific communities.
Conclusion: The Double-Edged Sword of Predictive Policing
Predictive policing holds promise for improving safety but presents significant ethical and practical risks. By reinforcing biases, targeting individuals unfairly, and eroding trust between law enforcement and communities, predictive policing may contribute to the very crime issues it seeks to prevent.
Without careful oversight, transparency, and community engagement, predictive policing risks becoming more of a tool for control than for safety. As society moves forward with AI, ensuring these technologies serve all communities equitably will be essential for a fair and just legal system.